What is Data Cleaning and Why is it Important? Exploring the Top 6 Data Challenges
Data cleaning is an essential process in data management, ensuring that the information used for analysis and decision-making is accurate, relevant, and reliable. In this blog post, we will discuss the importance of data cleaning and delve into the top 6 data challenges identified by Statista's 2024 research. We will also explore how NLSQL can help address some of these challenges and improve data quality for better insights and decision-making.
Statista 2024 Research Findings: Statista's 2024 research surveyed thousands of senior-level professionals in English-speaking countries to identify the top data challenges faced by organizations. The findings revealed the following top 6 problems:
1. Lack of analytical skills among employees (41% of respondents)
2. Data quality - bad, inaccurate, or redundant data (39% of respondents)
3. Managing compliance (39% of respondents)
4. Data democratisation - not everyone can access data on their own (36% of respondents)
5. Organisational silos - data is not shared among different groups (35% of respondents)
6. Data discovery - not knowing what data exists or who has what data (35% of respondents)
A bar chart illustrating these challenges will be available in the blog post for a visual representation of the findings.
The Importance of Data Cleaning: Data quality is a critical aspect of any data-driven organization, as it directly impacts the accuracy and reliability of insights derived from data. Poor data quality can lead to incorrect conclusions, misguided decision-making, and ultimately, a negative impact on the organization's performance. Regular data cleaning can significantly improve data quality, ensuring that insights from deep machine learning models and NLSQL are accurate and reliable.
NLSQL can help organizations overcome some of the top data challenges identified by Statista's research, specifically problems 1, 4, 5, and 6:
-> Lack of analytical skills: NLSQL allows users to query data using natural language, making it easier for employees with limited analytical skills to access and analyse data.
-> Data democratisation: By providing a user-friendly interface, NLSQL enables employees at all levels to access and analyse data independently, fostering data democratisation.
-> Organisational silos: NLSQL can facilitate data sharing and collaboration among different groups, breaking down organisational silos and promoting a data-driven culture.
-> Data discovery: NLSQL's intuitive search capabilities make it easier for users to discover and access relevant data, improving overall data visibility and utilisation.
Local Research on Data Quality: A local research study conducted among 120 data science experts from London meetups further emphasised the importance of data quality. Many NLSQL users employ data quality control and compliance-style questions with the NLSQL Teams bot daily to ensure data accuracy and relevance. This practice helps identify "wrong" categories after data synchronisations any anomalies or discrepancies in the data, enabling organisations to maintain high-quality data for improved insights and decision-making.
More:
Try NLSQL for 30 days
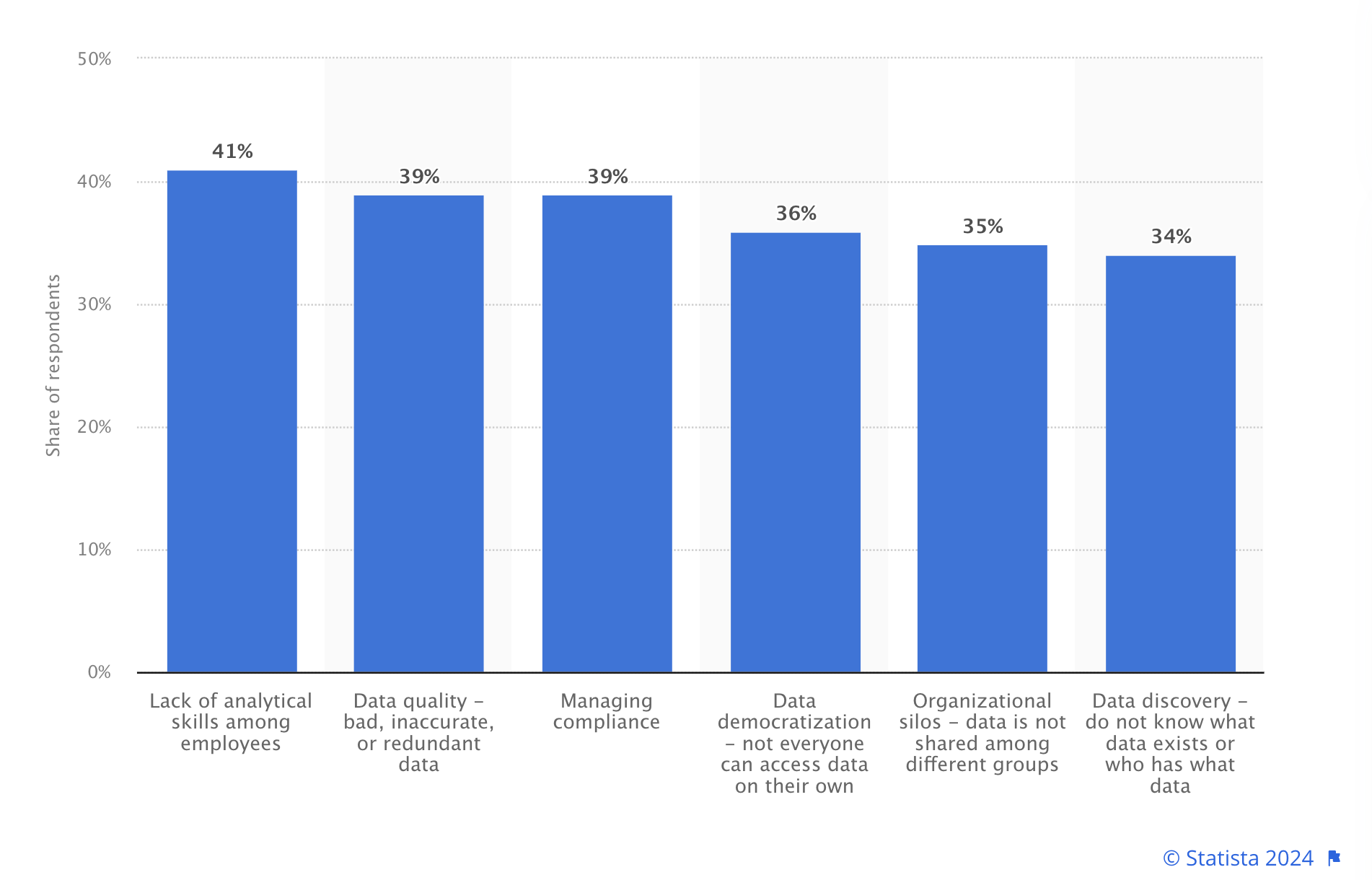
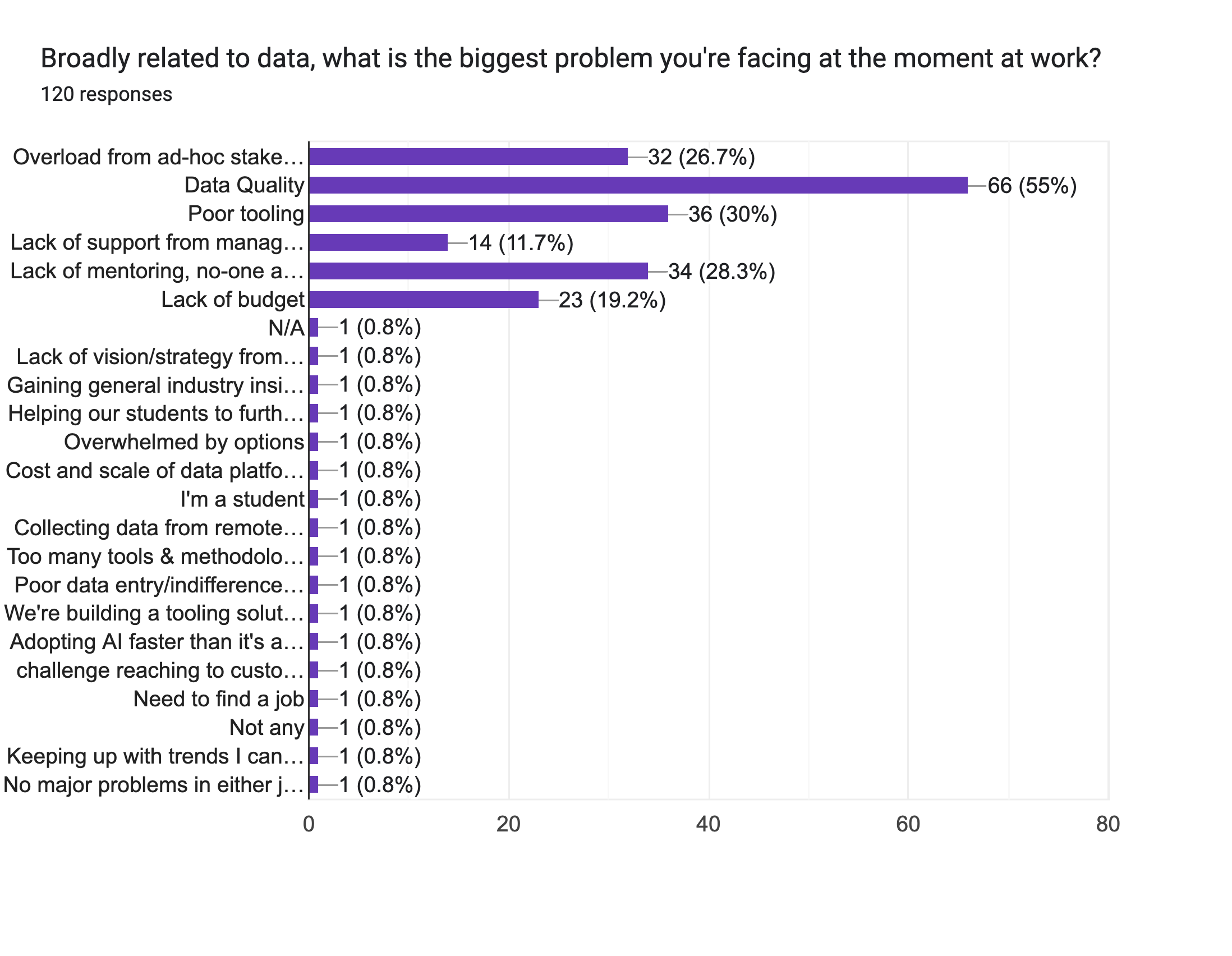